Decision trees are often used while implementing machine learning algorithms. The hierarchical structure of a decision tree leads us to the final outcome by traversing through the nodes of the tree. Each node consists of an attribute or feature which is further split into more nodes as we move down the tree.
But how do we decide which attribute/feature should be placed at the root node, and which features will act as internal nodes or leaf nodes? To decide this, and how to split the tree, we use splitting measures like Gini Index, Information Gain, etc.
In this blog, we will learn how the Gini Index can be used to split a decision tree. Before starting with the Gini Index, let us first understand what splitting is and what are the measures used to perform it.
What are splitting measures?
With more than one attribute taking part in the decision-making process, it is necessary to decide the relevance and importance of each of the attributes, thus placing the most relevant at the root node and further traversing down by splitting the nodes. As we move further down the tree, the level of impurity or uncertainty decreases, thus leading to a better classification or best split at every node. To decide the same, splitting measures such as Information Gain, Gini Index, etc. are used.
What is Information Gain?
Information Gain is used to determine which feature/attribute gives us the maximum information about a class. It is based on the concept of entropy, which is the degree of uncertainty, impurity or disorder. It aims to reduce the level of entropy starting from the root node to the leave nodes.
Formula for Entropy
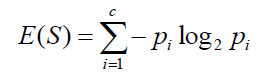
‘p’ denotes the probability, and E(S) denotes the entropy. Entropy is not preferred due to the ‘log’ function, as it increases the computational complexity.
What is Gini Index?
Gini index or Gini impurity measures the degree or probability of a particular variable being wrongly classified when it is randomly chosen. But what is actually meant by ‘impurity’? If all the elements belong to a single class, then it can be called pure. The degree of Gini index varies between 0 and 1, where 0 denotes that all elements belong to a certain class or if there exists only one class, and 1 denotes that the elements are randomly distributed across various classes. A Gini Index of 0.5 denotes equally distributed elements into some classes.
Formula for Gini Index
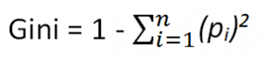
where pi is the probability of an object being classified to a particular class.
While building the decision tree, we would prefer choosing the attribute/feature with the least Gini index as the root node.
Stay tuned for the next installment in this series, in which Shagufta demonstrates Calculation of Gini Index for Trading Volume!
Disclosure: Interactive Brokers
Information posted on IBKR Campus that is provided by third-parties does NOT constitute a recommendation that you should contract for the services of that third party. Third-party participants who contribute to IBKR Campus are independent of Interactive Brokers and Interactive Brokers does not make any representations or warranties concerning the services offered, their past or future performance, or the accuracy of the information provided by the third party. Past performance is no guarantee of future results.
This material is from QuantInsti and is being posted with its permission. The views expressed in this material are solely those of the author and/or QuantInsti and Interactive Brokers is not endorsing or recommending any investment or trading discussed in the material. This material is not and should not be construed as an offer to buy or sell any security. It should not be construed as research or investment advice or a recommendation to buy, sell or hold any security or commodity. This material does not and is not intended to take into account the particular financial conditions, investment objectives or requirements of individual customers. Before acting on this material, you should consider whether it is suitable for your particular circumstances and, as necessary, seek professional advice.